Lifting the lid on AI: What affinity scores mean for marketers
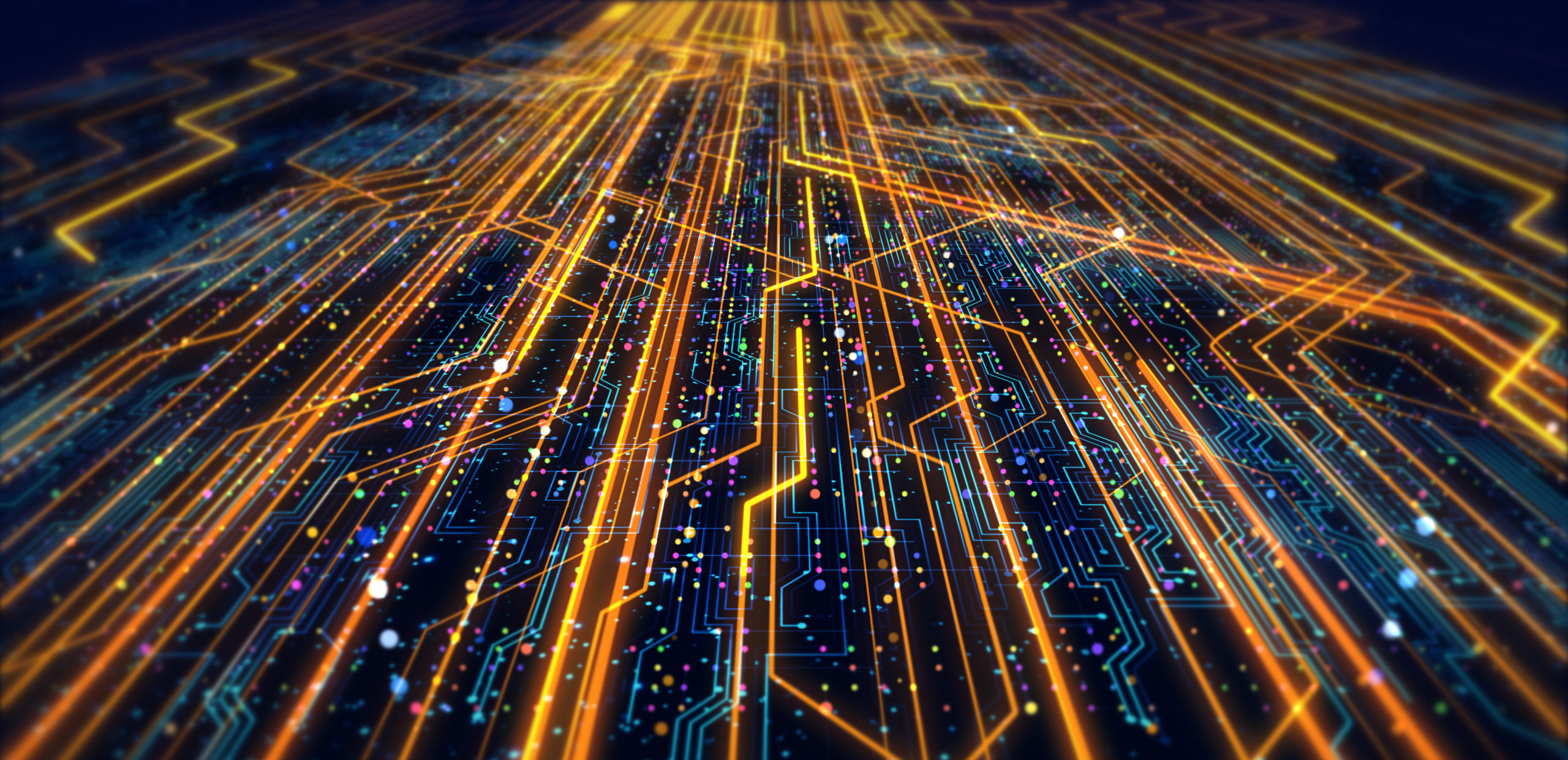
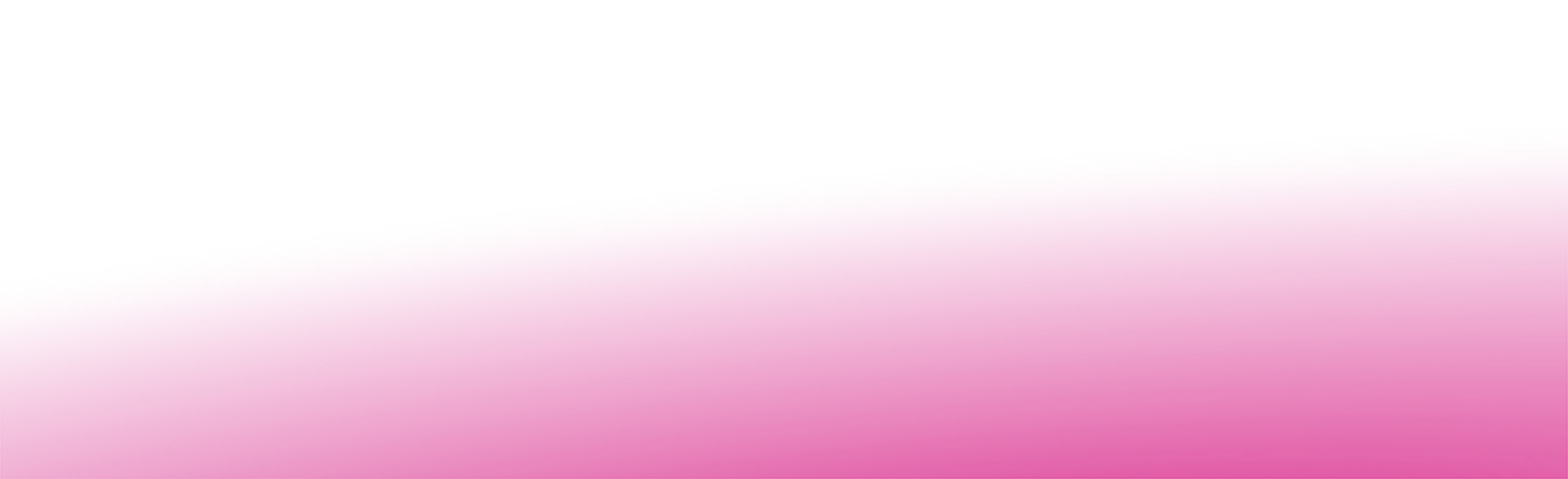
In a brick-and-mortar store, your best sales person is the one who knows their customer (that doesn’t mean knowing them personally, but rather aligning them to a typical customer type) and their product catalogue inside out. Machine-learning product recommendations not only replicate this experience online for your customers, but they can also do it at scale!
For example, our algorithms will be able to determine without any input from you that someone who has bought a tent and a sleeping bag is probably going camping. It will map that user journey against other similar user journeys and start to recommend products that are related to that path. As a marketer who knows their products, you could probably do this manually, but you couldn’t scale it.
Dotdigital allows you to simply drag-and-drop a block into place to enable this personalized recommendation for thousands of customers. In this blog, we chat with principle product manager Ian Pollard and product data scientist Sam Crawley to talk us though a recent innovation: product recommendation affinity scores, which are now available for you in Dotdigital.
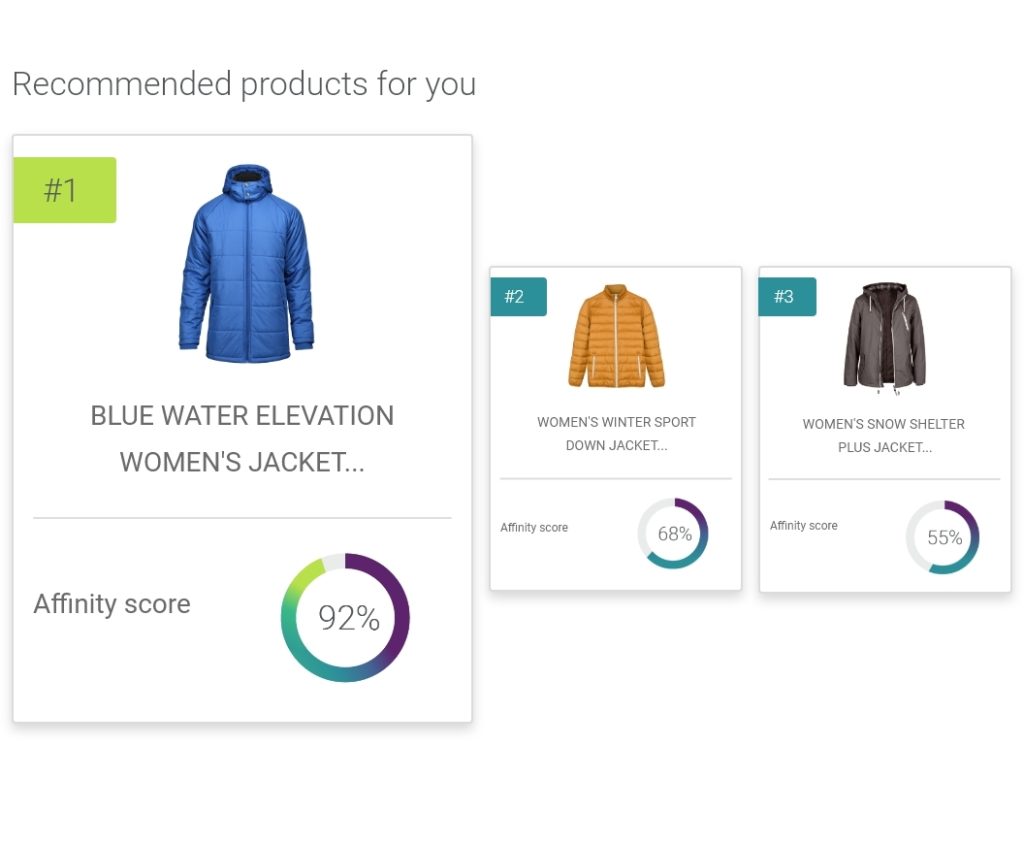
Who are affinity scores for?
Ian: Affinity scores are for curious marketers and merchants who want to understand a lot more about their customers. They uncover what kind of discrete customer/product relationships there are and also what are the strength of those.
We built our AI-powered product recommendations to allow merchants to create hyper-personalization and one-to-one content for their customers. All of the heavy data work is handled by the machine learning. The experience for our users is pretty much drag and drop. Drop this block into a marketing campaign – job done! What we then learned from our users is that they are incredibly curious and they want to understand what the AI is doing. That’s where affinity scores come into it, as they allow marketers to explore customers and the relationships they have with products; and visualize what AI says is a good fit. Excitingly, those same affinity scores will enable features coming up to power things like affinity-based auto-segments to find best contacts for a particular set of products. This would essentially automate segmentation.
Where did the need for affinity scores arise from?
Ian: AI traditionally is a black box. It’s only natural marketers then ask us: “How can I understand it?” and “What’s the AI doing?”. We wanted to enable that and open the black box up.
AIs are incredibly powerful and perform increasing functions within all aspects of business. It’s important to establish trust with the users of these AIs and one of the ways we do that is by visualizing the output for people – essentially letting people see what the algorithm sees.
Sam: It’s also important to note that the affinity scores have always been there, we just haven’t been surfacing them. So it’s not so much the need for affinity scores that is new, but the need to show them to our users and for them to make a judgment on how well a recommendation might perform.
How do they work?
Sam: As mentioned, affinity scores are not new, they are a by-product of our personalized recommendation algorithm, so the best place to start to explain them is there! When we run our personalized recommendations, we create a great big sudoku-style matrix between all contacts and all products. Like a sudoku, we prefill some of the squares with numbers we know, a contact’s preference score for each product they have ordered. This still leaves us with a huge amount of missing values in our ‘sudoku’! We tell the algorithm the rules and essentially let the algorithm attempt to solve the puzzle by filling in the missing numbers. These missing numbers are our predicted affinity scores, and for every contact we take the products with the top 10 affinity scores and serve them as recommendations for that contact.
We have two AI-powered product recommendation types in Dotdigital – lookalikes and best next. Lookalikes intake content-based analysis of the products themselves whereas best next examines similar shopper patterns. The affinity score will be unique to both the customer and recommendation type. The numbers indicate the level of confidence we have that the customer will have a preference for that product.
Were there any challenges that came up whilst developing affinity scores?
Sam: Well, trying to speak the same language as a computer is one! It’s a challenge we knew going in but the numbers spat out by our algorithm are meaningless on their own. The numbers it provides us with in the raw form are not percentages, just numbers without a hard-scale. So the main challenge is converting that number to a human readable format – or a percentage. We do that by reviewing what the highest possible confidence score is in the matrix and treat this as our 100% confidence, then scale every other number.
Could you explain the different tiers of score of what this would mean for an ecommerce marketer?
Affinity score | Product fit | Predicted outcome |
---|---|---|
70% to 100% | Highest level of confidence | This band has the highest predicted level of engagement, and therefore conversion. |
40% to 69% | Strong confidence | These products will have a strong fit for your contact, and the recommendations should drive both higher engagement and conversions for the contact. |
10% to 38% | Some confidence | The recommendations in this band should still see higher engagement than non-machine-learning recommendations. |
1% to 9% | Low confidence | Seeing this band score usually means that whilst there are some affinities which may result in higher engagement or conversion, we need to see more purchases to increase model accuracy. |
Ian: Any level of affinity you see in your account will be interesting. The higher the affinity score, the higher the confidence level. What that means in terms of outcomes for marketers, by using measures such as email click-throughs, length of web sessions, number of page views and ultimately the number of conversions, the higher the score, the more confident we are these would increase. Of course, the more orders the algorithm has to review from your account data, the more confident it will be in its predictions. Newer users or users who have only recently started syncing order data may see lower levels of confidence, but the algorithm is still picking up on data patterns and making informed predictions. The more data you feed it, the more likely you are to see scores in the higher brackets, but don’t be disheartened if you don’t see these scores at first.
Sam: You’re going to be seeing, at most, ten of these scores for product recommendations for a contact, and hopefully you’ll be seeing some or all of those in the top tiers. If you see a lot of them in the bottom two tiers, it could just mean there isn’t enough data for this particular contact to make a confident recommendation. The ‘cold-start’ problem is a known issue, (and one we see in real-life!) of what to recommend someone when you have no idea what they prefer. It’s fixed by feeding it more data. You have to remember, affinity scores are different from probability scores, they are confidence scores, so they are dependent on data.
Do you have any advice for ecommerce marketers who are going to use affinity scores?
Ian: If you’re not already using sample-sets of customers, then you should! Just as you might go and look at purchase behaviors like whether they have abandoned carts or gone to pages on your website, you should also, as part of that customer analysis, go and look at the products they are being recommended and what the affinities are for your products. I would then feed this back into your customer personas as part of your general understanding of your customer base.
Do you think AI-capabilities will become increasingly important for marketers?
Sam: Absolutely. The more data you have available, the more confident you can be about any decisions that are being made based on that data. But there’s an added layer of complexity to that. The more data you have, the harder it also is to put it to use. A marketer is just one person, and people, even a team of people, aren’t as good at sifting through large amounts of data as a machine. As we generate more data as a society and more people move online (especially during the pandemic), the need for AI will increase and marketers should start to leverage the capabilities now.
Ian: In addition to the time it will save you, naturally as humans, you start with a theory and you proceed. One of the issues with that is that your experience, the sum total of everything you know and everything you’re trying to do as a first step is potentially leading to a linear outcome. AI doesn’t come with your biases or prejudices, or expectations of what should happen. If there are patterns, it will find them, regardless of whether or not you think they should be there. It’s going to come out with something in some ways, more creative ideas that perhaps wouldn’t of occurred to you. Not just that, but it can do it at scale.
What does an ecommerce marketer need to start using affinity scores?
Ian: Simple, if you’re a customer, get in contact with your account manager. If you’re not and you’d like to learn more about our ecommerce offerings in general, get in touch and we’ll happily talk you through what we can do to help.